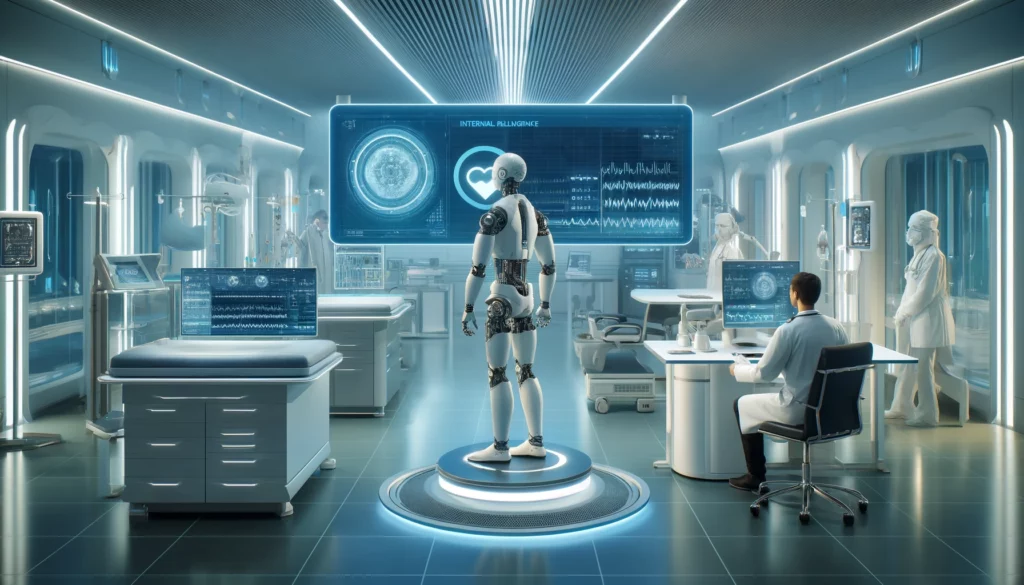
Artificial Intelligence (AI) is no longer just a futuristic concept—it’s a transformative force reshaping industries across the globe. From automated customer service to sophisticated data analysis, AI is proving its value as a powerful tool for enhancing efficiency and decision-making. In the realm of healthcare, its impact is particularly profound, offering new avenues for innovation in internal medicine and diagnostic practices.
In internal medicine, AI technologies are revolutionizing how physicians diagnose and treat diseases. With capabilities ranging from interpreting complex medical images to predicting patient outcomes, AI is not just assisting medical professionals but also enhancing the accuracy of their work. This integration of AI into medical diagnostics heralds a significant shift towards more personalized and timely patient care, which could redefine medical standards and outcomes.
This article aims to delve into how AI is altering the landscape of medical diagnostics. We will explore the myriad benefits that AI brings to the table, address the challenges and ethical considerations it raises, and provide insights into the future developments we might expect. By examining these facets, we aim to provide a comprehensive overview of AI’s role in modern medicine, highlighting its potential to transform patient care for the better.
Foundations of AI in Medicine
Artificial Intelligence (AI) and its subset, Machine Learning (ML), are transforming various fields, with medicine being one of the most impacted. Simply put, AI involves machines performing tasks that typically require human intelligence. Machine Learning is a specific approach within AI where algorithms learn from and make predictions based on data. This technological advancement offers an unprecedented ability to not only process vast amounts of data but also extract meaningful insights that can assist in clinical decisions.
In the medical field, several AI tools have become particularly influential. Neural networks, for instance, are a type of AI inspired by the human brain’s architecture, excellent at recognizing patterns in data. They are extensively used in diagnostic imaging, allowing for more precise interpretation of X-rays, MRIs, and CT scans than ever before. Another vital AI tool is Natural Language Processing (NLP), which enables computers to understand and process human language. In medicine, NLP is used to extract key patient information from unstructured data sources like doctor’s notes and clinical publications, helping to streamline patient care and enhance research.
Historically, AI’s application in medicine dates back to the 1970s with the development of early decision-support systems. One of the pioneering examples was the MYCIN system developed at Stanford University, which was designed to diagnose blood infections and recommend antibiotics. Although its use was primarily academic, MYCIN set the stage for the potential of AI in diagnosing and managing diseases. Another landmark was the introduction of the Health Evaluation through Logical Processing (HELP) system, which provided real-time decision support for various clinical conditions and was one of the first to be integrated into hospital information systems.
Today, these AI technologies are not just experimental but are integral parts of clinical practice, improving diagnostic accuracy, patient outcomes, and healthcare efficiency. As we continue to harness these powerful tools, the potential for AI in medicine is boundless, promising even greater advancements in the way we diagnose and treat patients.
Current AI Applications in Medical Diagnostics
The integration of Artificial Intelligence (AI) into medical diagnostics has revolutionized the way doctors detect and diagnose diseases. AI’s ability to quickly analyze and interpret medical data has led to significant advances in the identification and treatment of various conditions, ranging from cancer to cardiovascular and neurological disorders.
Cancer Detection and Diagnosis AI technologies have markedly improved cancer diagnostics. Machine learning models, particularly those using deep learning, can analyze pathology images with an accuracy that matches or even surpasses that of human pathologists. For example, AI algorithms are used in mammography and skin cancer screenings to detect subtle patterns that may elude the human eye. These tools not only increase the detection rates but also significantly reduce the time to diagnosis, which is critical for effective cancer treatment.
Cardiovascular Disease In the realm of cardiovascular health, AI applications have become essential for diagnosing heart diseases more accurately and swiftly. AI systems analyze echocardiograms to detect heart failure at stages far earlier than traditional methods might allow. These systems can predict heart attacks and strokes by identifying the telltale signs in heart imagery and physiological data points, thus providing a critical window for preventive strategies.
Neurological Disorders Neurological diagnostics have also benefited from AI, particularly in the early detection of diseases such as Alzheimer’s and Parkinson’s. AI algorithms process MRI scans to spot early signs of these neurodegenerative disorders long before clinical symptoms manifest significantly. This early detection is vital for managing the disease progression through timely intervention.
AI-Driven Diagnostic Imaging Tools One of the most impactful applications of AI in medicine is in diagnostic imaging. Automated radiology tools use AI to interpret X-rays, CT scans, and MRI images quickly and with high accuracy. These AI systems can highlight areas of concern that require a radiologist’s attention, thus optimizing the diagnostic process and reducing the workload on human specialists. This not only speeds up patient care but also improves diagnostic accuracy by minimizing human error.
Predictive Analytics for Chronic Diseases AI’s role extends beyond immediate diagnosis to the predictive analytics of chronic diseases. By analyzing patterns in historical patient data, AI models can forecast individual risks for diseases such as diabetes, chronic kidney disease, and more. This predictive capability allows for preemptive medical guidance and lifestyle adjustments to mitigate risk factors, illustrating AI’s proactive approach to healthcare.
Benefits of AI in Medical Diagnostics
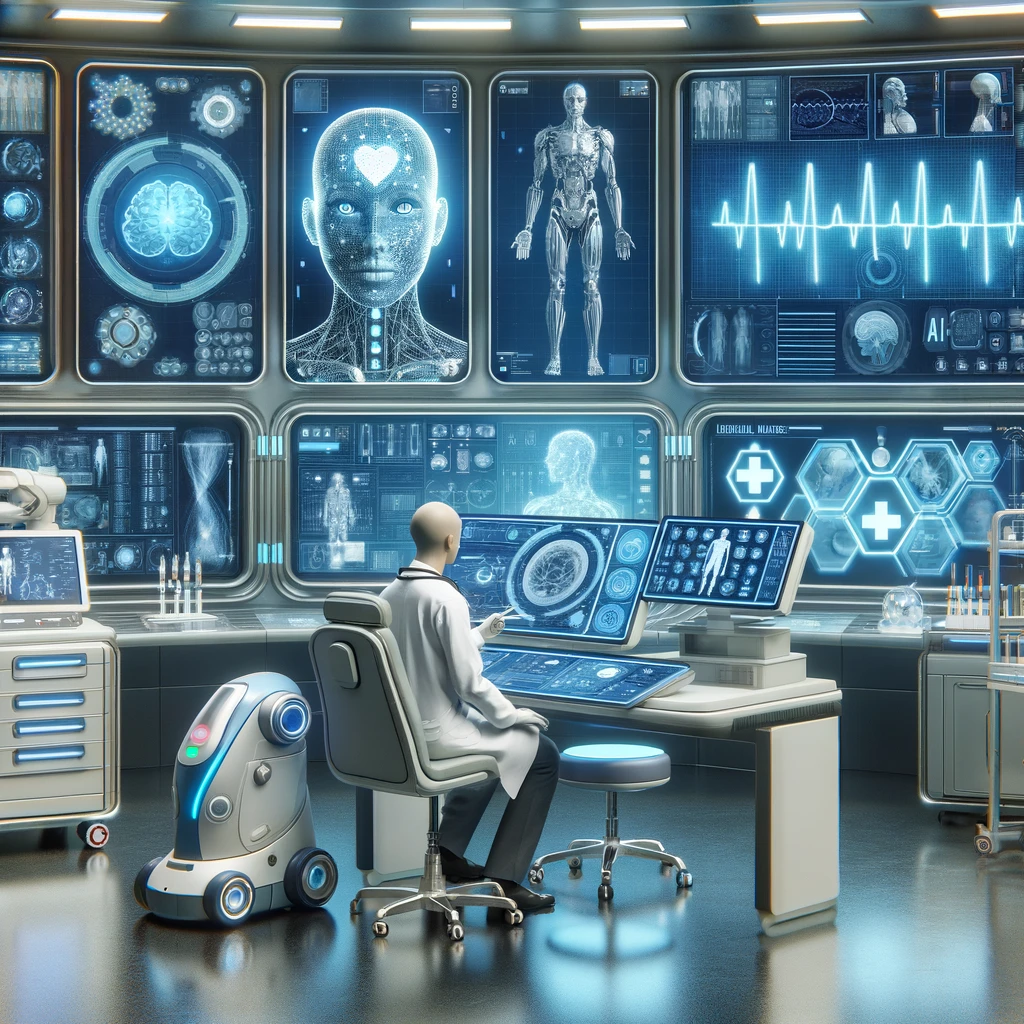
The application of Artificial Intelligence (AI) in medical diagnostics has brought about transformative benefits that are reshaping patient care. From improving diagnostic accuracy to customizing treatment plans, AI is enhancing the capabilities of medical professionals and the outcomes for patients. Here’s a closer look at some of these key benefits.
Increased Precision and Speed of Diagnostics AI’s ability to quickly process and analyze medical data enhances both the precision and speed of diagnoses. For instance, AI-driven algorithms applied in radiology can detect abnormalities in imaging faster than human radiologists, with studies showing that AI can reduce reading time by up to 50% while maintaining or improving accuracy. This rapid, precise analysis means quicker diagnoses, leading to faster treatment decisions and better patient outcomes.
Handling Large Volumes of Data Modern medicine generates vast amounts of data, from clinical records and lab results to imaging studies. AI excels at managing these large datasets, extracting relevant insights that might go unnoticed by human observers. For example, AI systems can integrate data from various sources to give a comprehensive view of a patient’s health, predicting potential health issues before they become evident through traditional methods.
Personalization of Medical Treatment AI contributes significantly to the personalization of medical treatment. Predictive algorithms analyze patient data to forecast health trajectories and suggest interventions tailored to individual patients. This approach is particularly beneficial in managing chronic diseases, where AI can help in crafting personalized treatment plans that adjust to the changing health conditions of patients, thus optimizing therapeutic effectiveness.
Reduction of Workloads and Human Errors By automating routine tasks, AI allows healthcare providers to focus more on patient care rather than administrative duties. This automation helps reduce the workload on clinicians, which can decrease burnout and improve the quality of care. Furthermore, AI reduces the risk of human errors in diagnosis. For example, AI systems provide a second layer of review for diagnostic imaging, catching potential errors or oversights by the human radiologist, which enhances patient safety and diagnostic reliability.
Ethical Challenges and Considerations
While the integration of Artificial Intelligence (AI) in medical diagnostics offers numerous benefits, it also presents several challenges and ethical considerations that need careful management. These issues are crucial to ensuring that AI contributes positively without compromising patient trust or care quality.
Privacy and Security of Medical Data The use of AI in medicine involves handling sensitive patient data, raising significant privacy and security concerns. Protecting this data from breaches is paramount, as leaks can have serious repercussions for patients’ privacy and the trustworthiness of medical institutions. AI systems must be designed with robust security measures, and data handling must comply with legal standards such as HIPAA in the United States. Continuous monitoring and updating of security protocols is essential to guard against evolving cyber threats.
Algorithmic Bias and Demographic Precision AI models can only be as good as the data they are trained on. If the training data is biased or lacks diversity, the AI’s diagnostic recommendations may also be biased. This can lead to poorer health outcomes for underrepresented groups. For example, an AI trained predominantly on data from one ethnic group may be less accurate when diagnosing conditions in people from a different ethnic background. Addressing this requires a concerted effort to use diverse training datasets and to continuously evaluate and adjust AI models to ensure they treat all demographic groups equitably.
Dependence on Technology and Loss of Traditional Diagnostic Skills There is a concern that increasing reliance on AI could lead to a degradation of traditional diagnostic skills among healthcare providers. As AI tools provide more diagnostic support, there is a risk that medical professionals may become overly dependent on technology, potentially leading to skills decay. This could be detrimental in situations where AI is unavailable or fails. Balancing AI use with the ongoing training and skill development of medical professionals is critical to mitigate this risk.
Regulations and Ethical Standards in the Use of AI The rapid development and implementation of AI in medicine have outpaced the establishment of specific regulations and ethical guidelines. Ensuring ethical AI use involves addressing issues such as patient consent for using their data, transparency about AI’s role in diagnosis, and accountability for AI-driven decisions. Developing comprehensive regulations and standards that guide AI implementation in medical settings is essential to fostering an ethical environment that safeguards patient interests while encouraging innovation.
Case Studies and Recent Developments
The application of Artificial Intelligence (AI) in medical diagnostics has been marked by numerous success stories, cutting-edge innovations, and collaborative ventures that highlight the potential and effectiveness of AI technologies. These developments not only demonstrate AI’s capabilities but also pave the way for future advancements.
Relevant Case Studies One notable example is the use of AI in diagnosing diabetic retinopathy, a leading cause of blindness. Google Health developed an AI system that analyzes eye scan images to detect this condition at an accuracy level that matches top-rated human ophthalmologists. This system is already in use in clinics in India, providing critical diagnostic support in areas with a shortage of specialists.
Another impactful case study involves the use of AI at the Mayo Clinic to predict cardiac events. An AI algorithm developed through this program can analyze electrocardiograms to predict the likelihood of irregular heart conditions that might not be detected by a standard interpretation. This tool has proven to be a valuable asset in preventive cardiac care, potentially saving lives through early intervention.
Recent Innovations and Key Research Findings Recent innovations in AI have included the development of AI systems capable of processing and interpreting large datasets to predict patient outcomes across several conditions. For example, IBM’s Watson for Health has been able to predict with high accuracy which patients are at risk of developing congestive heart failure, up to months before they exhibit any symptoms.
Furthermore, recent studies have shown that AI can significantly improve the accuracy of breast cancer detection. Researchers at MIT and Harvard developed an AI model that reduces false positives and false negatives in breast cancer screening, providing a more reliable diagnostic tool than traditional methods.
Collaborations Between Medical and Technological Institutions The success of AI in medicine is often fueled by collaborations that bridge the gap between medical knowledge and technological prowess. A prime example of such a partnership is the collaboration between Microsoft and Novartis, focusing on using AI to transform medicine by developing solutions that can scale across different countries and healthcare systems. This partnership aims to innovate in ways that individual institutions working alone could not achieve.
Another significant collaboration is between NVIDIA and the National Institutes of Health (NIH) in the United States. They are working together to enhance the use of AI in clinical research, particularly in improving the detection of diseases such as cancer through better imaging technologies.
Future of AI in Medical Diagnostics
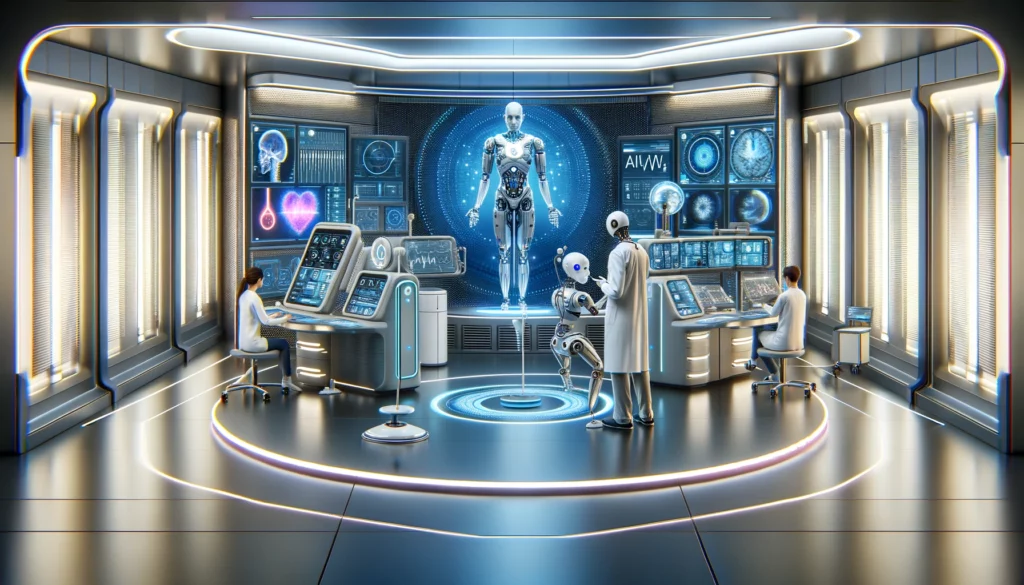
Emerging Trends in AI and Medicine The landscape of AI in medicine is continuously evolving, with several promising trends on the horizon. Self-learning AI systems represent a significant leap forward. These systems, which improve their algorithms based on new data without human intervention, are starting to emerge. This advancement could lead to more adaptive and precise diagnostic tools that evolve with the latest medical research and data trends.
Another major trend is the integration of AI into personal medical devices. Wearable health monitors, such as smartwatches that can detect heart rates and predict heart events, are already in use. The future points towards devices that could diagnose more complex conditions autonomously by analyzing data collected directly from the user’s environment and body, providing real-time health monitoring and alerts.
Expert Perspectives on the Future of AI in Diagnostics Experts in both technology and medicine are optimistic about the potential of AI to transform diagnostics further. Many believe AI will become a standard assistant in diagnostic procedures, providing second opinions and identifying patterns that may not be visible to human eyes. For instance, leaders in neurology anticipate AI systems that could predict neurological degenerations like Alzheimer’s disease long before the first clinical symptoms appear.
Additionally, there is a consensus that AI could democratize healthcare by making high-level diagnostic tools available in remote or under-resourced areas, thereby improving healthcare accessibility and equity globally. However, experts also caution about the ethical implications and the need for clear guidelines to manage AI integration responsibly.
Potential Impact on Medical Training and Clinical Practices The rise of AI is set to have a profound impact on medical training and practice. Medical education may need to evolve to include more interdisciplinary studies that combine data science, ethics, and AI technology with traditional medical training. Future medical professionals might need to be as proficient in managing AI systems as they are in patient care, emphasizing the role of AI as a tool rather than a replacement for human judgment.
In clinical practice, AI is expected to shift the focus from routine diagnostics to more complex case management and personalized medicine. It could enable doctors to spend more time on patient interaction and care management, enhancing the quality of care and patient satisfaction.
Conclusion
Throughout this discussion, we have explored the transformative impact of Artificial Intelligence (AI) on medical diagnostics. From increasing the precision and speed of diagnoses to handling vast data sets and personalizing medical treatments, AI’s benefits are profound. However, these advancements do not come without challenges. Concerns around privacy and security, the potential for algorithmic bias, and the necessity of maintaining traditional diagnostic skills amid increasing technological reliance highlight the complexity of AI integration.
The reflection on the balance between technological innovation and human care is critical. While AI can process data and identify patterns at an unparalleled speed and scale, it lacks the human touch—empathy, ethical judgment, and the nuanced understanding of patient needs that are vital to holistic care. Thus, while we embrace the efficiencies and capabilities of AI, we must also reinforce the irreplaceable value of human interaction in healthcare.
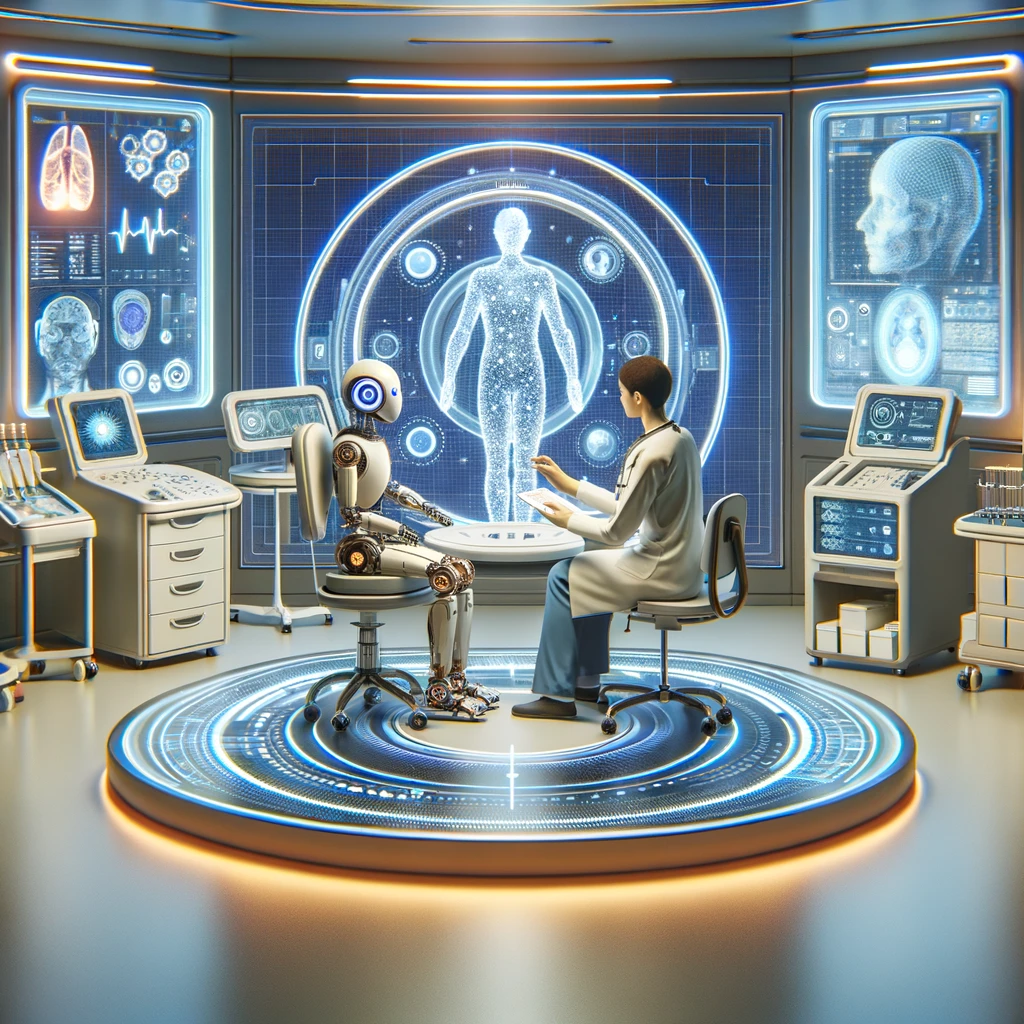
Looking ahead, the call for ongoing critical evaluation and adaptation to new technologies is clear. As AI continues to evolve, so too should our strategies for integrating it into healthcare practices. This includes updating medical training to incorporate AI knowledge, creating robust ethical guidelines to govern AI use, and ensuring that technology enhances rather than replaces the human elements of medical care.
By maintaining this vigilant approach, we can harness the full potential of AI to not only innovate diagnostics but also elevate the standard and accessibility of medical care worldwide, ensuring that technology acts as a complement to, not a replacement for, skilled human providers.